The 21st century is witnessing a remarkable transformation in technology and innovation with the advent of Generative AI. While traditional AI in financial services has been around for decades, Generative AI has taken center stage, creating transformative impact.
This revolutionary technology has not only democratized access to unmatched productivity but has also unveiled previously untapped potential. Numerous exciting use cases have emerged from next-generation customer service solutions to enhanced financial planning, fraud detection, and more.
The clear market leader is OpenAI, as its GPT models are helping reset the way businesses interact with their data, their work, and even their customers. However, Google, Facebook, and the lesser-known Anthropic have released their own generative AI models. Equally, generative AI comes with unique risk factors – like hallucinations and data privacy – that senior leaders should be aware of before fully committing to the technology. In this article, we’re sharing our vision for the future of AI-enabled financial services and exploring the top six use cases set to make their mark in the industry. We’ll also examine some best practices for companies looking to integrate generative AI into their day-to-day operations.
How financial services are adopting generative AI
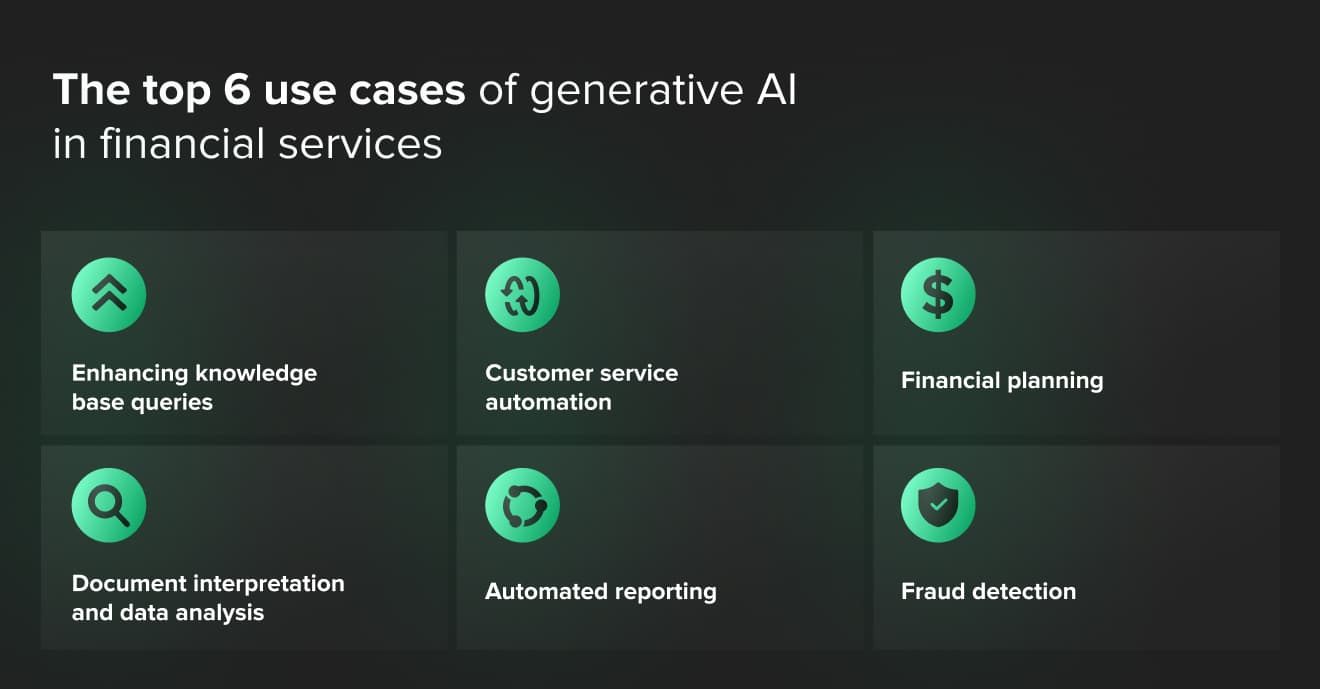
Enhancing knowledge base queries
Generative AI models can provide a chat interface that understands search queries and responds using human-like text. Microsoft’s Bing and Google’s Bard currently offer this functionality, while financial services firms have also begun exploring this use case using their proprietary data. For example, Morgan Stanley’s partnership with OpenAI is helping financial advisors search the firm’s vast knowledge base of investment strategies, market research, and analyst insights more easily.
Financial advisors are able to access a personalized learning environment that provides the most up-to-date information on market opportunities and how to meet them with Morgan Stanley products and services. As a result, the firm’s financial advisors are investing more time in client relations and generating revenue by applying analysts’ insights.
Customer service automation
Financial institutions can use client-facing generative AI programs to enhance their customer service with their ability to adapt to digital and financial literacy for both support agents and the users they serve, offer AI-to-human support, and build trust with customers.
Generative AI is able to adjust to different levels of financial/digital literacy. Able to modify its output to meet different needs and automate repetitive or routine workloads, it can remove account creation and management touchpoints for support agents, who are then free to focus on specialist problems like debt recovery and insurance claims. Plus, banks could help their customers understand notable trends in their transaction history and become more financially literate too.
Where support is concerned, customers can gain the support they need through application processes via the delivery of timely product recommendations based on account data, credit history, and live interactions. While this has been frequent in already established AI like Azure Cognitive Services for years, there’s an expectation that generative AI will only enhance the personalization process.
What’s more, resolving issues quickly and efficiently through the use of generative AI will build trust with customers. The removal of both digital and human touch points throughout application processes will make for frictionless service and human intervention only when necessary. The result: customer trust and satisfaction.
Financial planning
Generative AI technologies are shaking up various areas of the financial services sector, not least, in budgeting and wealth management. By leveraging open banking and generative AI technologies, banks and lenders can now categorize customer financial data and spending habits more accurately. Similarly, lenders could assess applicants’ loan eligibility more precisely, using markers to establish creditworthiness and generate new revenues with products, such as flexible repayments, that are tailored to a customer’s risk profile.
Likewise, recent developments in generative AI software are democratizing AI-enabled trading and have opened the door to a new style of wealth management, motivating a shift from traditional trading to round-the-clock neobrokerage that is accessible to a broader customer base. For one, Bloomberg recently revealed its own large language model, BloombergGPT, trained on their database of financial documents from the last forty years and designed to guide investment strategies. BloombergGPT outperformed more generalized generative AI programs, but newly released plug-ins to OpenAI’s GPT, PortfolioPilot, show similar promise. Regardless, AI-enabled software can help customers take advantage of market opportunities based on their financial goals and risk appetite – something worth noting for financial companies that want to stay competitive with their offerings.
Document interpretation and data analysis
Generative AI has also initiated a transformation within the RegTech industry by reinventing the process of document analysis. One example is via FiscalNote, a ChatGPT add-on aimed at enhancing the software’s performance on legal and regulatory tasks. The plug-in provides real-time access to legal, political, and regulatory data. As a result, it deepens ChatGPT’s policy knowledge base and helps companies respond to potential gaps in their compliance posture and adjust their risk accordingly.
The generative AI solution, therefore, ensures companies can navigate dynamic market conditions with more confidence as well as save time and resources on managing their liability. Long-term, this means generative AI could help senior leaders provide more predictable shareholder returns by hedging against risk more effectively.
Automated reporting
Furthermore, generative AI is streamlining the analysis and reporting of other data types, for instance, in accounting or expense management. By automating analysis processes, senior business leaders are saving time, reducing human error, and improving the quality of their reports. In turn, financial services firms are boosting their growth and enhancing their digital journey via stronger, data-driven corporate strategies.
Fraud detection
Finally, GPT models could improve existing fraud detection solutions, for example, by enriching transaction data and monitoring new areas of customer activity. This is because, while companies have spent years fine-tuning existing solutions to tackle this problem, AI models are now advanced enough to offer new types of value.
Specifically, generative AI could take over currently under-explored areas of fraud analysis. Already, Stripe has partnered with OpenAI to detect cybercriminals using customer forums to obtain sensitive user data. Equally, generative AI could enhance the customer verification process by creating unique security prompts for customers to use while logging in.
The realities and limitations of adopting generative AI in financial services
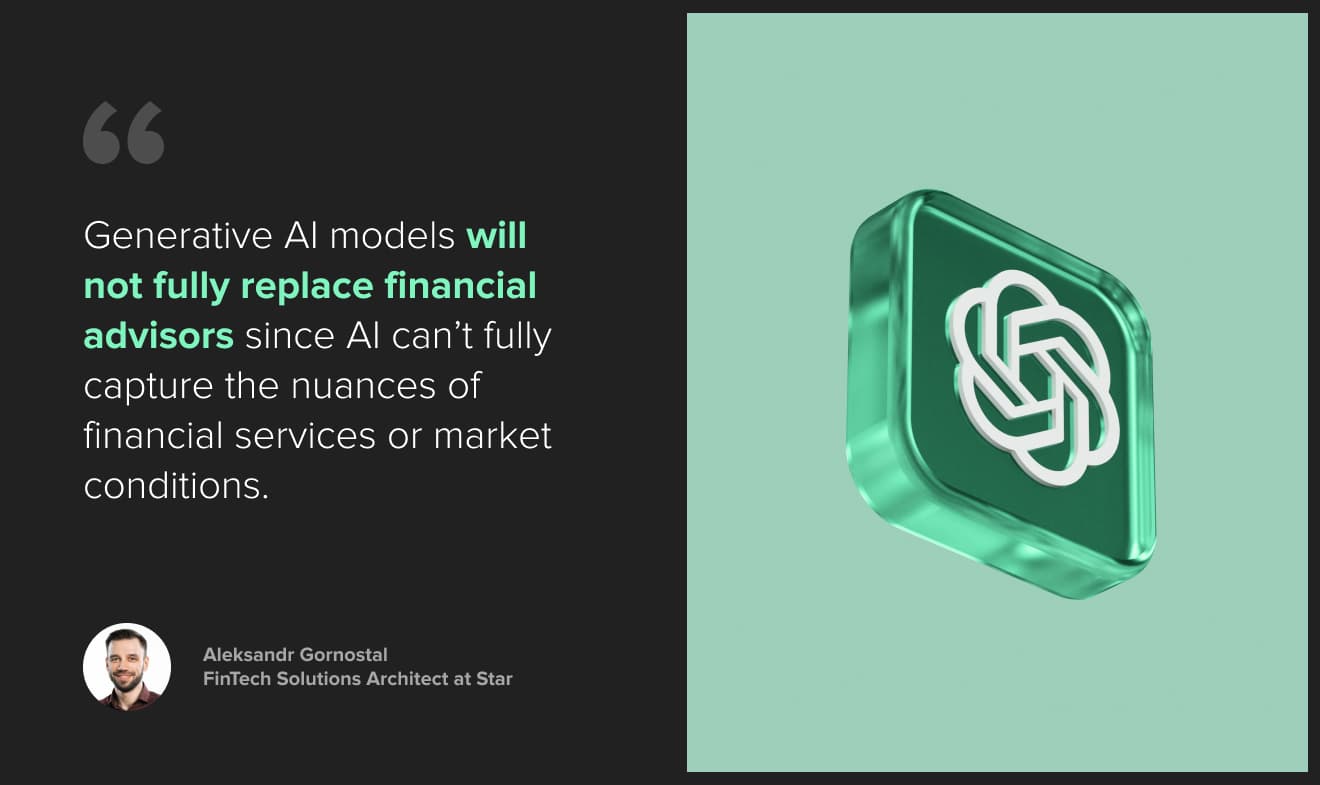
It’s important to note that generative AI has its limitations, risk factors, and a best practice methodology for adoption. First of all, generative AI models will not fully replace financial advisors since AI can’t fully capture the nuances of financial services or market conditions. For instance, a client may still see value in a company despite trading algorithms predicting a fall in its stock price. While trust can be built through the combined use of generative AI support and human interaction, it can be undone by system failures or machine inaccuracies.
Secondly, training data sets can create performance issues through biased results. Therefore, ongoing supervision, guidance, and feedback are vital to accelerate digital journeys and enhance employees’ performance and customer trust. Similarly, generative AI models are prone to hallucinations (where the program ‘makes up’ facts or sources). As a solution, limiting your model’s ‘temperature’ (how ‘creative’ it is) can help mitigate this and ensure that what is received is wholly accurate information.
Finally, business leaders must always protect proprietary company information and customer data. So, external collaboration with experienced third-party tech consultants or agencies can help you adopt AI securely while also accessing expert implementation support at the same time.
How to adopt generative AI effectively
Generative AI is a rapidly evolving technology, and we’re already seeing large language models like OpenAI GPT being utilized in service offerings, to enhance AI-human support and customer service experiences.
At this early stage, practice and experimentation within a risk-free environment are essential to truly understanding the limitations – in particular, how different departments within an organization each experience these limitations and how different generative AI tools perform.
Ultimately, businesses need to ensure their employees understand the fundamentals of data security and customer privacy, so they can use generative AI safely without risking their compliance posture. Then, after the training period, they can engage with employees on what AI-enabled processes work best in their organization, for example, identifying the optimal configuration settings to achieve desired outputs; in which departments it should be utilized or avoided within the business; and where to leverage new AI features or plug-ins as they become available.
Harness AI to drive meaningful value
At Star, we identify optimal business use cases, effectively integrate generative AI layers while ensuring the highest level of cybersecurity and regulatory compliance, and deliver remarkable commercial returns that reveal hidden pockets of value for you and your customers.
So, whether you’re looking to start your digital journey or enhance your existing processes, we can help. Our in-house experts can help you launch products that ‘wow’ customers every time.