When faced with a technically involved project, how do you get it from the concept stage to a solution in action? That’s one of the significant challenges facing developers at every level, particularly those in the auto industry who need to understand driver behavior. Recently, Star’s Head of Solution Architecture Oleg Lebedyn and Head of Design Manuel Gattinger collaborated with CloudMade’s CTO, James Brown and Head of Data Science, Dr. Pavel Negadailov to discuss how to rise to the challenge viewing a personalized driving coach project as an example.
To find out more in-depth detail regarding the full-stack, scalable services Star provides, watch the full webinar with Star and CloudMade experts.
Beginning with the endgame in mind
At the heart of every viable idea must be a goal. For a personalized driving coach, the concept begins by asking, “Is it possible to support drivers to maintain a smooth driving style that combines efficiency with the ultimate goal of safety?” In the ideation process, foundational information is established such as the audience being catered to, the scope of the project, where the collected data can be found, and the pain points that will be addressed.
Is an aware driver seeking to improve fuel efficiency? Can you coach a new driver to navigate the streets safer? Is it possible to provide relevant, valuable information to experienced drivers? It all begins by setting accurate expectations at the start.
Tackle the design challenges
During creation, the product goes through several checkpoints before being built out. The main challenge is to find a solution that affects change in the user’s habits. As a personalized driving coach is meant to be a consistent engagement point intended to improve their driving over time, the user must find the content ‘evergreen’. Yet as a solution that’s presented in-vehicle to a driver or on their device, it’s imperative that it does not become a distraction, living in the background.
Since the driving coach is likely to find a home with a vast range of demographics, the results have to be intuitive and easily digested by the driver. Determining how to detect problematic or unsafe driving habits and encouraging how to correct them is clearly an objective across the complete lifecycle.
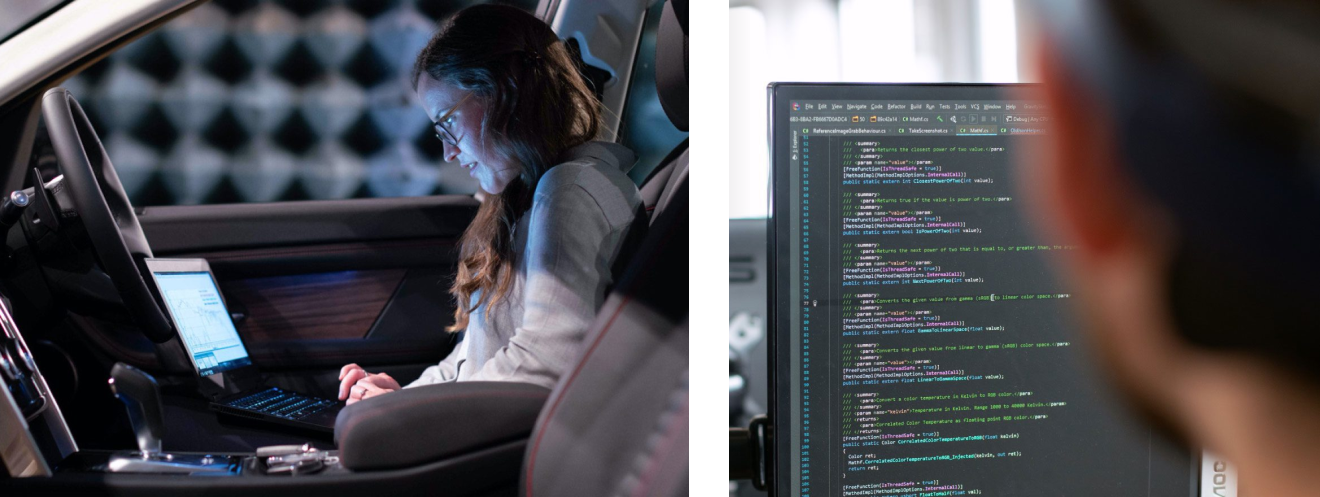
Engineer with intention
Design is further complicated when a cross-device ecosystem is introduced, massive amounts of data are introduced, and the information must be transmitted outside the vehicle, requiring data security. A robust offline mode is essential along with a continuous learning model, both of which center around machine learning.
Cornerstone content includes a data collection platform, intelligent ML developments, broad-scope integration, a gamification aspect, and validation.
Phases of development
The research phase
Research is foundational to accomplishing the defined goals, involving extensive competitor and user research. User personas help to flesh out the user journey and fill in the blanks, from initial introduction to the personalized driving coach and throughout their lifecycle with the vehicle.
The design phase
Taking into consideration the brand’s ethos and appearance, the UI environment is crafted to be cohesive across the user’s devices, whether the driver information center in-vehicle, their smartphone, or otherwise. Design starts with use cases, progresses to wireframes, then visual design prior to being engineered for vehicle implementation. The key to every stage of the design process is continuous, robust testing that verifies every facet that’s implemented.
The machine learning architecture phase
For this type of system, the technical framework is hybridized to synchronize in-vehicle tech with cloud-based learning. This mix is ideal with all the machine learning performed in the cloud to prevent the need for using vast amounts of computational power - resources the vehicle must share with other applications. Cloud-based data also enables the personalized driving coach to aggregate behavioral information from other drivers for cohort learning.
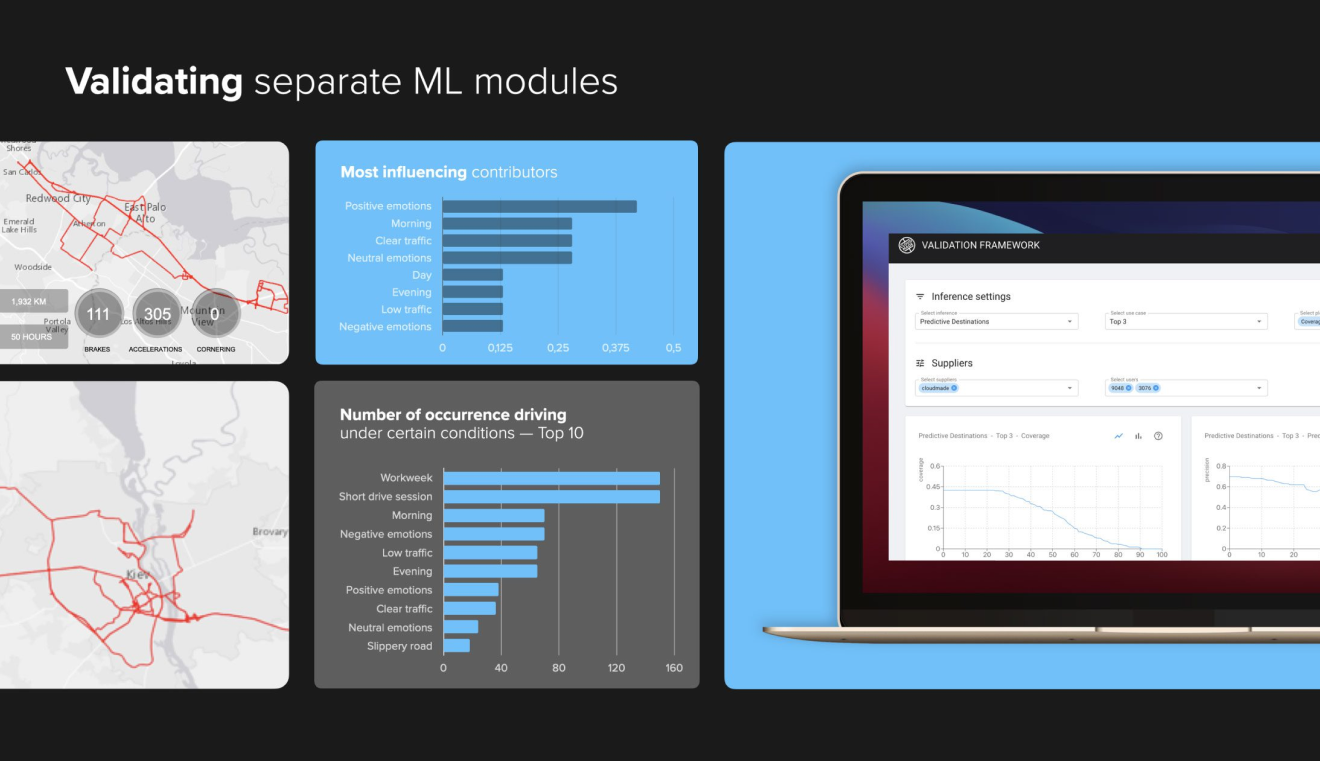
The validation phase
To the client, three aspects that are most important for this type of solution:
- Does it work accurately? Is user behavior predicted correctly and are the predicted destinations accurate?
- Are the users achieving their objectives? Is changing driving behavior identifiable with the product? Is the driver enjoying and engaging with the personalized driving coach?
- Are there fewer dangerous events by the driver? The ultimate goal is to achieve safer drivers with fewer incidents on the roads.
These three criteria are used to validate the solution and refine it at the end-to-end testing stage as well as post-production launch.
Summary
Whether it’s reducing the frequency of road collisions, influencing driver behavior, or developing more convenient features and solutions, it requires a team of specialists to carry an idea to implementation. Star’s services such as intentional consultation, strategy, development, as well as deliberate partnerships will help you achieve your objectives in the automotive space.
What does it take to bring your concept to life? It requires the ability to identify an audience, understand the challenges, and define a development process through four critical stages: research, design, architecture, and validation.