Last year, IBM sold Watson Health. The AI healthcare technology project focused on bringing world-class care to every corner of the world. As investments and acquisitions in the sector proliferate, it can seem surprising IBM has scaled back and sold many of the healthcare components of its AI business division to investors. What happened?
There's no denying AI's incredible potential to transform healthcare. Nonetheless, Watson suffered many issues. It was far from the quick answer machine that people saw on the Jeopardy! game show. Watson struggled to adapt to new electronic health systems, understand doctor's notes and patient histories. It also didn't help that IBM was targeting one of the highest bars in modern medicine – real-time cancer diagnosis.
Ultimately, Watson’s moonshot ambitions may have been too steep. It was often plagued by complexity and gaps in the data. Watson excelled at ingesting vast amounts of research from medical papers. It could then point to treatments physicians may have missed.
Unfortunately, however, it lacked flexibility and universal applicability and IBM’s ambition to support global treatments was untenable. For instance, applying sample data extracted from people in New York to inform decision-making for populations in China did not lead to the expected outcomes.
The fundamental demographics of healthcare don't scale to the challenges in front of us. We can only address them through technology and by improving efficiency, focus and outcomes.
IBM's experience with Watson is a lesson to heed. You can have the right technology, but you need to understand how it connects to users and how to deploy it effectively and specifically across targeted areas.
AI is no longer a bleeding edge. It is already here and improving care delivery. As you move to incorporate or expand AI into your MedTech and digital healthcare products, you should be integrating these five essential recommendations into your strategy.
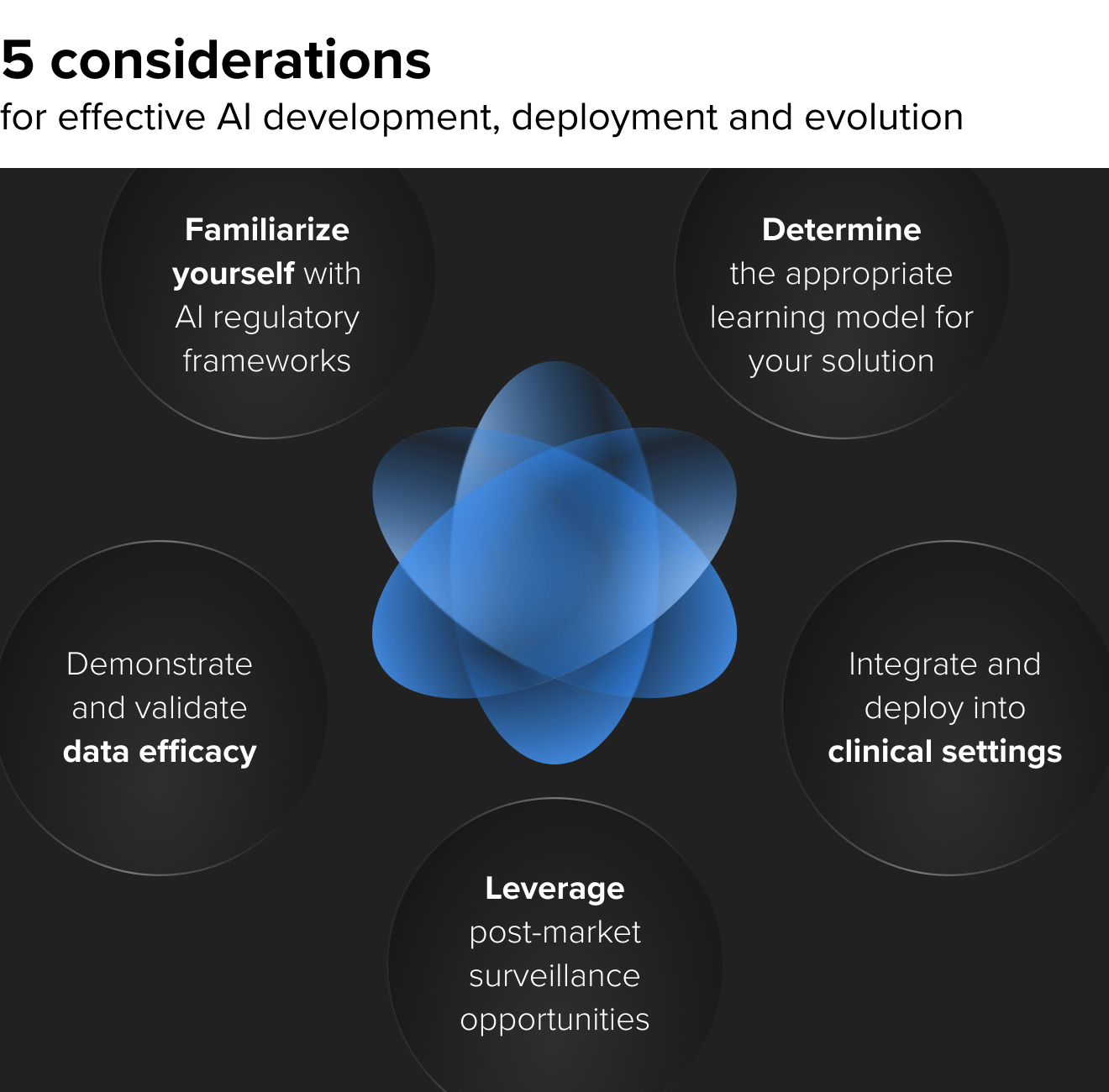
1. AI regulatory frameworks are helping streamline approval
Whether you decide to certify your device or not, it's important to consider existing and soon-to-arrive regulations around AI technology, especially within the context of SaMD product development. The FDA and the EU are solidifying frameworks that will help streamline and simplify the approval process while considering the technology's highly iterative nature.
The FDA, for example, already has policies specifically for 510(k) software modifications that focus on user risk resulting from software change and now outlines categories that may require premarket submissions, such as changes to risk controls or alterations that affect clinical functionality or performance.
Moves like these signal the FDA and other regulatory agencies' prioritization of approving more AI/ML and other novel healthcare technologies that are rapidly growing each year.
Concurrently, gaining approval is an equally beneficial business opportunity. It not only distinguishes your product and helps build credibility with payers and providers but is also a barrier to market entry and payer support for your competitors.
2. Determine whether a continuous learning or static model is the right solution
Learning models are the foundation of artificial intelligence. An early question you'll need to answer is whether you'll focus on a continuous learning or static model for your healthcare algorithm. Each has its own advantages.
Learn core AI use cases in healthcare and what you need to get the best ROI
Static models can be less expensive to deploy, provided you have the necessary data in place. They are also easier to manage because you don't have to create pathways to feed new data since the data will always stay the same. If the model's accuracy is 97%, for example, that's where it will remain regardless of how much data you generate until you build another algorithm – although that might have to go through the regulatory approval process again.
Continuous learning models can offer greater adaptability and are useful when you have limited amounts of initial sample data. Ideally, they get better, and accuracy increases over time. In addition, if the algorithm learns by itself and improves, you don't necessarily have to seek regulatory approval for every iteration.
The primary downside is that continuous learning models could also get worse. Your original data may have had a bias or come from a skewed sampling – reinforcing how critical data quality and training are.
There's no one-size approach. While continuous models get the most spotlight nowadays, they are still uncommon in healthcare due to their increased complexity and added risks. You must focus specifically on the healthcare context
in which your solution will be deployed, the data you have available and what approach best fits the end users they trust and use.
3. Demonstrating efficacy on different data sets when selling into new domains
You cannot overestimate the importance of data in ML development. How you label and train it is critical. However, many early-stage HealthTech companies run into a chicken and egg problem.
How do you generate data and demonstrate its efficacy for a device that has not been clinically approved yet, especially if you don’t have a historical device from which you can gather data from a non-AI-related instance? Or, in some cases, you might have data, but the data used to generate the AI models wasn’t yours. This is why testing and validation are essential.
Furthermore, you need to consider the demographics within your data. Perhaps the product was tested on a group of people in a certain geography, and you’d like to take your product to national or international markets. We’ve seen this with our clients who needed to re-validate their product because it was approved for the EU market but required clinical trials that included US citizens in order to put it on the US market, and this was despite both sample groups possessing similar characteristics.
Beyond this, you need to understand if there are gaps in your data sets that can bias or influence the algorithm. Your model only has the information you've fed into it, so it can erroneously perceive that events can't happen just because data is missing, ultimately affecting its credibility and quality.
AI in radiology is one example. If you deploy an algorithm at a particular site, it will work with data from there. X-rays and other scans are largely universal in how they are performed. Still, slight variations in technique or equipment can result in your machine learning solution attaining a different output.
For these reasons, as you scale your product and even begin its trial launch, validation exercises are vital for confirming AI efficacy in the target environment.
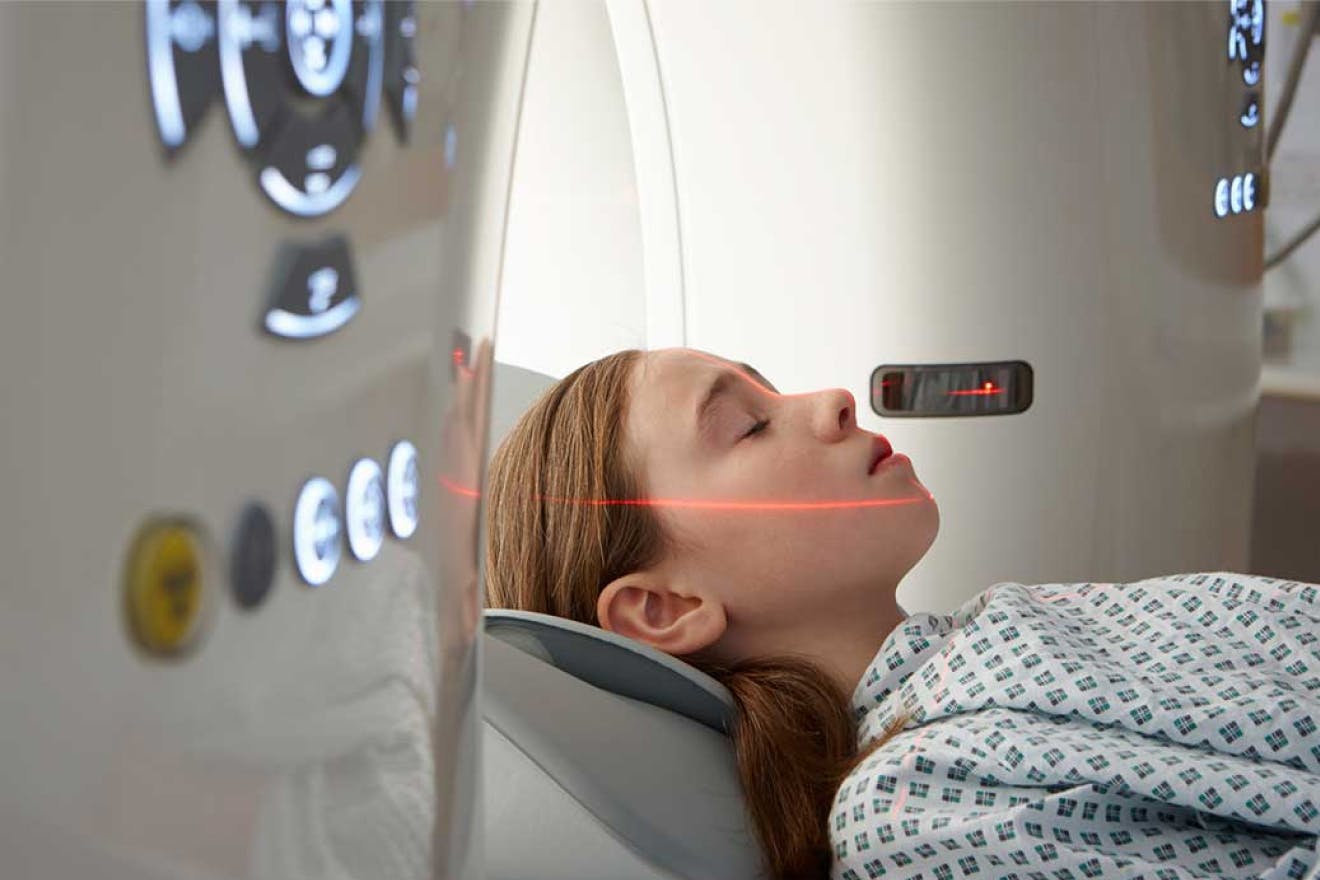
4. Integration and deployment into clinical settings
Despite all the investment and research, most clinical AI models, unfortunately, don't get used. Building an initial model on a small data set is not that hard. But rolling it out, deploying it and getting it used is another chasm that hinders AI use in healthcare.
Having an AI model doesn't solve anything. It's only when it gets into the clinical workflow that it becomes effective. This is a different challenge to building an AI model. Its usability and interoperability in a clinical environment must be addressed with your solution.
We know healthcare professionals are under immense stress, have incredibly busy days, work at short-staffed facilities and consequently, often burnt out. That's why AI is necessary, and we need to consider this as we build technology solutions.
Ask yourself these questions as you build your product:
- How does my product connect and interact with real people and users?
- How does it disrupt workflows (even if it’s for the better)?
- How do we show efficacy and validate AI works on their data?
- How do you surface information so it is meaningful, consumable and trustworthy?
- Where does the device send data, and how does this data interact with people?
- What kind of change management is needed when introducing my AI?
Radiology is a great example as it's already proven to be one of the most successful incorporations of AI into healthcare. In the past, radiologists would analyze a scan, provide an assessment and attach it to an image. Now, we've introduced an algorithm that does the evaluation. The radiologist may still review the evaluation or just a certain sampling of images with a low confidence score, but it's not the same queue as in the past.
How has this impacted not only the radiologist's workflow but other connected healthcare professionals as well as the patient? What happens if something goes wrong and the system needs repairs that will take a few days?
Considerations like these are vital as you think about how your product will interact with different stakeholders, helping ensure your product will be adopted and used in the long run.
5. Post-market surveillance
Post-market surveillance for machine learning in healthcare is not just confirming your product is working. It’s an important learning opportunity to observe how your solution functions in the real world. At this stage, you must carefully watch and learn from other successes and failures in the industry.
We need to monitor the device to ensure it's operating within its guardrails and, of course, that it is safe and effective. Simultaneously, we must analyze the industry, academic literature and your competitors during this stage. There are key learning opportunities here for you to identify potential hazards and impacts that could potentially present a risk to the way your algorithm is working.
In short, it's a chance to improve your product as it continues its life on the market.
Develop relevant, effective and impactful healthcare AI
AI will play an increasingly important role in healthcare throughout the next decade. We're already seeing more and more healthcare algorithms being approved and deployed in the market. Nonetheless, you must think beyond the algorithm and understand how it functions as part of a larger solution. That includes the considerations discussed in this article and much more, from collecting data to deploying and integrating it into clinical workflow, which can be potentially disruptive and organizational change management.
Finally, remember the entire lifecycle of your MedTech products. Think about the post-market opportunities and monitor them in the context of the state-of-the-art as you begin updates and product evolution.
Ensure the success of your investment into healthcare AI solutions and work toward safety, efficacy and improved health. Incorporate these strategies into your solution roadmap from day one.